Re-thinking Statistical Testing in Sports Science Research: The Case for Single-Subject Designs
- John R. Harry, PhD, CSCS
- Mar 5, 2021
- 5 min read
Updated: Mar 26, 2021
I'm going to start this post by saying that I am in no way a statistics expert. I have my little toolbox of group-focused statistical tests I was taught how to conduct, and I tend to stay in my lane and use those tests in my research to avoid looking like a fool. I think many others in sports science employ a similar strategy, which can complicate things when trying to learn about how athletes perform or respond to an intervention. One thing that was beaten into my brain by some of my formal and informal doctoral mentors (mainly Dr. Janet Dufek and Dr. Barry Bates) is the value of the replicated single-subject approach. I think it's relatively clear that this approach has some situational advantages when compared to the group-design approaches we are almost exclusive taught in undergraduate and graduate programs.
The main reason I, along with some other 'young' colleagues (e.g., Dr. Jeff Eggleston), feel as though we need to "re-think" statistical test selection relates to the basic "generalize then analyze" approach of commonplace group statistical tests (e.g., t-test, ANOVA, etc.). Without question, there are situations where it's valuable to understand whether the "average" athlete will respond to an intervention in a certain way. However, no two athletes are the same, or even all that similar to be honest. This might seem odd for me to say because humans have very similar neuro-musculo-skeletal structures and general functional capacities. However, each athlete has a unique set of past experiences, environmental constraints, and mechanical abilities from which the athlete "learns" (i.e., gains a new perception of the requisite demands) after each exposure to a movement task or intervention. As a result, the data obtained from an individual will have a fair amount of variability (i.e., intra-subject), which can affect statistical power. This is why those who have me as a peer-reviewer often get a comment requesting justification for the number of trials recorded per athlete during a testing session (this blog is going to make me an easy to identify reviewer in double-blind review processes!). When this is combined with the other unique qualities of each athlete, it's reasonable to expect a fair amount of variability among athletes (i.e., inter-subject), which can also affect statistical power and lead to erroneous results.
What all this means is that we should not typically expect each athlete to perform similarly to the "average" athlete emphasized in typical group-based statistical designs. Instead, we might consider replicated single-subject designs, where each athlete's performance or response to an intervention is recorded and an inferential assessment is conducted. Then, the inferential results of each athlete's performance or response is generalized (i.e., the single-subject protocol is replicated). What this approach does is flip the "generalize then analyze" group approach to an "analyze then generalize" approach that has tremendous potential to reveal previously masked responses from each athlete. For those interested in the underpinnings of replicated single-subject approaches, I've attached below a copy of a chapter written by Drs. Barry Bates, Roger James, and Janet Dufek for Dr. Nick Stergiou's book titled "Innovative Analyses of Human Movement," which details how the replicated single-subject approach deals with common statistical assumptions and what not.
Let's use some data to highlight the masked responses that could otherwise be useful for a scientist or practitioner to know. The table below provides individual and group summarized results for countermovement vertical jump time (i.e., time to takeoff) as measured in seconds from the onset of the countermovement through the instant of takeoff. I chose this variable because it's a nice jump strategy metric associated with jump explosiveness, but the selected variable for this example is arbitrary. The data for each participant represents the mean across 8 recorded trials before (pre-training) and after (post-training) an 8-week resistance training program (details of the training are described here). The sample consisted of 25 active females. I'll first use a simple group inferential approach that is appropriate for this type of pre-post comparison (paired-samples t-test).
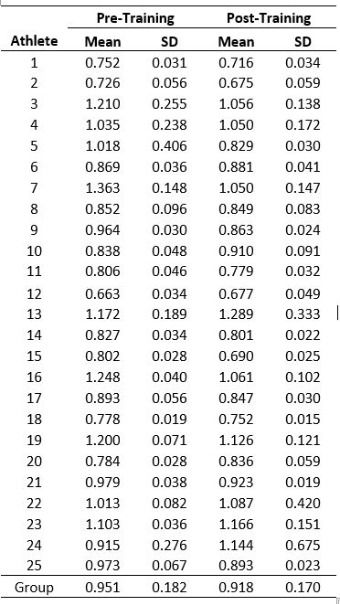
The results of the group-focused paired samples t-test indicated a non-significant difference in jump time pre-to-post training (one-tailed p = 0.072; two-tailed p = 0.145) where the magnitude of the difference was trivial in size (Cohen's d = 0.19). From this statistical test, it could be concluded that the resistance training program was not effective in altering the quickness of the vertical jump for the average female from this sample (i.e., the group did not respond to the treatment for jump time). Now, we could simply increase the sample size until we achieve a "statistical difference", but that's kind of cheating, and most sports scientists can't go get more athletes than they have on their roster.
I can feel your intrigue as you think, "would we get the same result if we took a replicated single-subject approach?"
Well, luckily for you I have the answer! For this analysis, I used the Model Statistic, which is described in detail in the book chapter I referenced previously. In short, the Model Statistic is similar to a t-test, but it accounts for the standard deviations for the comparative mean values in addition to the number of trials used to calculate the comparative means and standard deviations (the number of trials also determines the critical value used to determine the whether the test returns a 'significant' or 'non-significant' outcome). The results of the replicated single-subject approach indicated that 9 of the 25 participants (i.e., ~36 percent!) demonstrated a significant difference (p < 0.05) between pre- and post-training tests (participants 1, 7, 9, 15, 16, 18 20, 21, and 25 if you're wondering), with each decreasing jump time (i.e., they became quicker). Moreover, the magnitudes of the differences (i.e., the Cohen's d) for those participants ranged from 1.2 to 4.5 (large to super-monstrous depending on the interpretation scale you use). I think most sports science researchers and practitioners would find this to be a very informative result that could surely be used to make actionable decisions for their athletes that would otherwise not be possible if relying on the group-based approach.
So, am I saying we need to throw out group analyses all together and focus solely on the replicated single-subject approach? No. Not at all. So don't reference this post claiming that I do (I will Liam Neeson you from Taken if you do...).

Instead, scientists and practitioners might consider incorporating both group and replicated single-subject analyses to consider the results more holistically. This might help with interpretations and making actionable decisions for a group of athletes or even just a few from a sample, which could really make a difference for a practitioner! When it comes down to it, the question in need of an answer has to be the primary driver for the inferential approach we take. Far too often we choose the quick and easy approach rather than the best approach for what's at hand. Hopefully, this post will spark some thought and discourse among scientists and practitioners to keep moving the field forward. The computer is telling me this is a 5-minute read, so I will stop here before I bore you into not finishing it!
See you next week!
Comments